Introduction of Machine Learning Testing
In our ever-rapidly changing technological world, ML represents a breakthrough, bringing change to areas such as healthcare and finance. As these intelligent systems become more integrated into our daily operations, it is important to ensure their quality and performance remain consistent through extensive testing This is where machine learning testing comes in, indicating a major shift in the quality assurance field.
Traditionally, quality assurance (QA) in programming has involved cautious manual testing and pre-planning automated tests to ensure applications run correctly. However, with the development of AI models, the amount of information dealt with is so small, and traditional QA strategies are not. ML systems develop over time, making decisions based on patterns learned from data rather than strict rules. This automatic characteristic of ML frameworks requires creative display in testing techniques, is adaptable, and is complete to the point of tending to the complexities of AI.
Understanding Machine Learning Testing
Machine learning testing extends beyond determining whether a piece of software works as intended. It explores the approval of the information, model, and review strategy. The goal is to ensure that the ML model makes accurate, unbiased predictions or decisions that are capable of dealing with new and inconspicuous data. This includes several key tests, such as information approval, model approval, and execution testing.
Data validation ensures that the data used to train and test an ML model is high quality, accurate, and free of any prejudice that may be bias the results. It also informs people to be careful. Performance testing evaluates a model’s accuracy, speed, and ability to generalize from training data to predict new data.
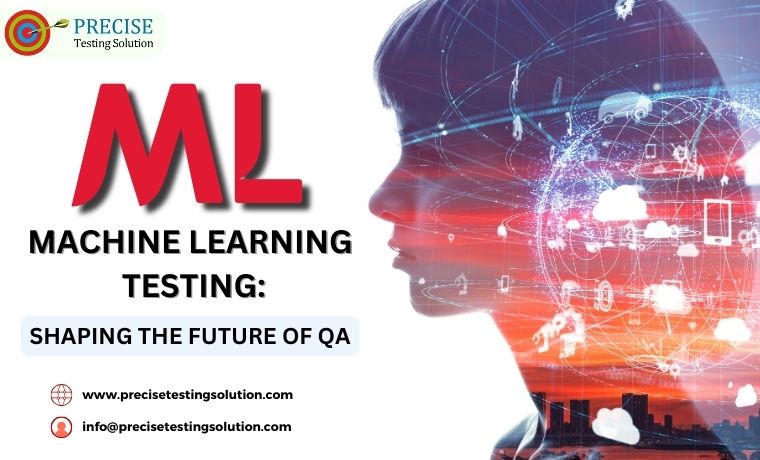
The Challenges of Machine Learning Testing
Quite possibly, the main test in ML testing is the sparsity of a reasonable “right response” for each circumstance. ML models typically deal with probability and uncertainty, which ways that experimenters must win now probabilistic reasoning and recognize that the goal is not perfection but rather to minimize error within winning limits.
Additionally, ML models are susceptible to the biases of the training data, which can result in skewed or inappropriate results. This is in relatedness to traditional software, where results are predictable and easy to verify versus expected results. Distinguishing and decreasing these predispositions is a significant piece of ML testing, which requires a profound comprehension of the information and the setting where the model works.
The Role of Automation and Continuous Testing
Automation is important in ML testing because of the amount of data added and the need for continuous analysis as models learn and evolve. Automated testing frameworks and tools have been created to address the unique requirements of ML systems. The CI/CD pipeline is enabled. This ensures that prototypes are thoroughly tested and validated throughout their entire lifecycle, from initial development to deployment and beyond.
Ethical Considerations and Accountability
As ML processes involve multiple decision-making activities, ethical considerations and accountability become more important. ML testing should include ethical audits and compliance checks to ensure that samples do not introduce bias or cause issues. This includes not only technical assessments but also ethical assessments and governance frameworks to help guide the development and implementation of machine learning systems.
The Future of Quality Assurance in ML
The tools and techniques used to evaluate machine learning are also evolving. We can distinguish progressed strategies for deciphering and deciphering model ways of behaving, which will help in testing and approving complex models. In addition, incorporating artificial intelligence (AI) into the testing processes themselves can additionally improve the quality and productivity of ML frameworks.
Conclusion
In conclusion, machine learning testing is reshaping the future of quality assurance, bringing new challenges and opportunities. This requires a shift from traditional testing methodologies to a more dynamic, data-driven approach. As we move into this new terrain, our main goal will be to build a robust testing framework that can ensure, and ultimately deliver, the reliability, accuracy and efficiency of machine learning algorithms confidence and trust have emerged in AI technology.
For more information, visit our website at www.precisetestingsolution.com or call our office @ 0120-3683602. Also, you can send us an email at info@precisetestingsolution.com
We look forward to helping you!
How to Identify Email Spoofing Attempts
What Is The History Of Spoofing Spoofing, in the
A Comprehensive Guide to Optimizing Your A/B Testing
What is A/B testing? Also known as split A/B